Editor's Note: Take a look at our featured best practice, Enterprise Data Management and Governance (30-slide PowerPoint presentation). Unleashing the Potential of Enterprise Data Management: Navigating the Data Deluge In today's fast-paced digital landscape, enterprises are witnessing an unprecedented surge in data generation year after year. As reported by Grand View Research, the data management solutions market attained a [read more]
The Impact of Data Modeling on Data Quality and Integrity
Also, if you are interested in becoming an expert on Digital Transformation, take a look at Flevy's Digital Transformation Frameworks offering here. This is a curated collection of best practice frameworks based on the thought leadership of leading consulting firms, academics, and recognized subject matter experts. By learning and applying these concepts, you can you stay ahead of the curve. Full details here.
* * * *
At present, data-driven enterprises require effective data management to remain competitive with their rivals. Enterprise solution architects need to comprehend how data modeling impacts data quality and integrity for optimal results. Understanding this subject matter is not just beneficial, it is vital. This post will explore some of the complexities surrounding data modeling while emphasizing its significance in making sure organizations manage their information with accuracy and integrity.
At the conclusion of this article, you will gain valuable insights into various aspects of data modeling. In particular, its role in improving data quality and strategies for protecting enterprise-scale databases.
Data Modeling Plays an Essential Role in Improving Data Quality
Data quality is of utmost importance for enterprises seeking to make informed decisions. Poor data can lead to incorrect analysis, misguided strategies, and, ultimately, financial losses. An effective data modeling program lays the foundation for increasing data quality by creating clear definitions, data types, and relationships between datasets.
An organized approach to data modeling ensures data is accurate, consistent, and relevant. By clearly outlining data attributes and their acceptable formats, organizations can reduce risks of data entry errors and inconsistencies. For example, when creating customer databases using, this model will specify formats for phone numbers, addresses, and email IDs to create uniformity among records.
Data validation is an integral component of maintaining data quality, and effective data models facilitate this process. By including validation rules within their models, enterprises can automatically check for data integrity as new entries enter the system. This ensures that only high-quality information is stored in databases for analysis.
For instance, in a sales database, data models may include constraints to guarantee that sales figures cannot go negative, thus improving trust in the data and increasing reliability for analytics and reporting initiatives.
Data quality requires continuous management and refining. Well-designed data models enable enterprises to constantly assess and improve the quality of their data over time, using key performance indicators (KPIs) related to data quality for tracking metrics such as completeness, accuracy, and timeliness.
Regular reviews of data quality metrics enable enterprises to identify trends, anomalies, and opportunities for improvement in the data model. With this as their starting point, solution architects can then implement strategies to address any identified issues and strengthen a culture of data-driven decision-making.
Assuring Data Integrity through Robust Modeling
Data integrity is vital to reliable business operations and sound decision-making, and robust data modeling plays a central role by outlining clear structures and rules for data management. Entity integrity ensures each record in a database is uniquely identifiable (typically enforced with primary keys), while referential integrity maintains accurate relationships among tables via foreign keys.
Robust data models can also feature additional constraints and checks, such as unique constraints and check constraints, to prevent duplicate or invalid data entries. Organizations can systematically enforce business rules and workflow requirements by implementing well-defined constraints. For instance, ensuring no duplicate email addresses exist in customer databases to avoid issues regarding communications and identity verification.
Robust models also facilitate the use of triggers and stored procedures to automate data integrity tasks, with triggers taking the form of specific actions being automatically taken when certain conditions are met, such as updating a record when its counterpart changes in another table. This level of automation reduces human error while guaranteeing consistent and reliable data across your database system.
Emerging Trends in Data Modeling
As data continues to proliferate at an unprecedented pace, evolving data modeling trends impact how organizations utilize, analyze, and leverage their assets. One such trend is NoSQL databases’ rapid adoption. This is designed specifically to manage large volumes of unstructured data more effectively than traditional relational databases. Their flexible schema design makes NoSQL appealing to businesses that must handle varied types of information at scale quickly and rapidly.
Another prominent trend is the integration of machine learning and artificial intelligence into data modeling processes. These technologies offer predictive analytics, automated anomaly detection, and advanced pattern recognition. This provides deeper insights and enhanced decision-making abilities. Machine learning models can dynamically adjust schemas according to changing data landscapes for optimized performance.
Cloud-based data platforms also mark an important shift. Offering scalable infrastructure and advanced tools that streamline data integration and management, hybrid and multi-cloud environments enable businesses to adapt rapidly to changing customer demands while taking advantage of an array of services and capabilities.
Preparing for the Future
As organizations transition towards becoming data-driven environments, it becomes crucial for them to adopt an aggressive data management and analytics approach. Preparing for such an eventuality involves strategic planning, technological investment, and skills development. This includes setting up an infrastructure capable of securely handling large volumes of information efficiently and safely. You can invest in scalable storage solutions, real-time processing capabilities, and advanced analytics tools.
Fostering a culture focused on data within an organization is integral. Employees at all levels should receive training on data literacy to ensure they know how to interpret and use it for decision-making, supporting the wider adoption of data-driven practices across all departments.
Staying abreast of technological innovations is also essential to maintaining a competitive advantage. New innovations such as blockchain for data security, augmented analytics to gain deeper insights, and edge computing for faster processing at source could have profound effects on how data is managed and utilized by an organization. Organizations should explore and adopt such innovations for themselves to keep pace with industry innovations.
Focusing on ethical data practices and meeting regulatory standards cannot be overemphasized. With data governance becoming ever tighter and demands for privacy increasing, implementing robust governance frameworks and transparent data practices are integral parts of maintaining trust as well as regulatory compliance.
Conclusion
Modernizing data infrastructure should be seen as an imperative in today’s data-rich environment. By investing in scalable and secure storage solutions, promoting data literacy initiatives within their organization, and adhering to ethical data practices, organizations can build a solid base that facilitates innovation and expansion. Integrating emerging technologies, like blockchain, augmented analytics, and edge computing will further expand the ability to manage and utilize data effectively. A proactive approach to data management will not only ensure compliance and security but also create opportunities for business intelligence analysis and strategic decision-making.
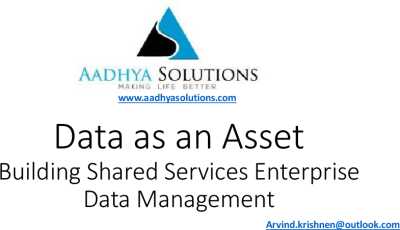
Want to Achieve Excellence in Digital Transformation?
Gain the knowledge and develop the expertise to become an expert in Digital Transformation. Our frameworks are based on the thought leadership of leading consulting firms, academics, and recognized subject matter experts. Click here for full details.
Digital Transformation is being embraced by organizations of all sizes across most industries. In the Digital Age today, technology creates new opportunities and fundamentally transforms businesses in all aspects—operations, business models, strategies. It not only enables the business, but also drives its growth and can be a source of Competitive Advantage.
For many industries, COVID-19 has accelerated the timeline for Digital Transformation Programs by multiple years. Digital Transformation has become a necessity. Now, to survive in the Low Touch Economy—characterized by social distancing and a minimization of in-person activities—organizations must go digital. This includes offering digital solutions for both employees (e.g. Remote Work, Virtual Teams, Enterprise Cloud, etc.) and customers (e.g. E-commerce, Social Media, Mobile Apps, etc.).
Learn about our Digital Transformation Best Practice Frameworks here.
Readers of This Article Are Interested in These Resources
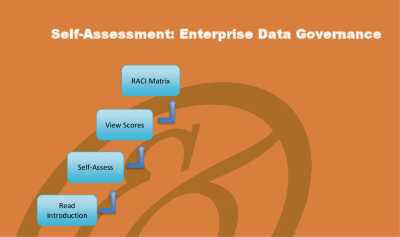
|
24-slide PowerPoint presentation
|
|
21-slide PowerPoint presentation
| |||
About Shane Avron
Shane Avron is a freelance writer, specializing in business, general management, enterprise software, and digital technologies. In addition to Flevy, Shane's articles have appeared in Huffington Post, Forbes Magazine, among other business journals.Top 10 Recommended Documents on Data Governance
» View more resources Data Governance here.
» View the Top 100 Best Practices on Flevy.
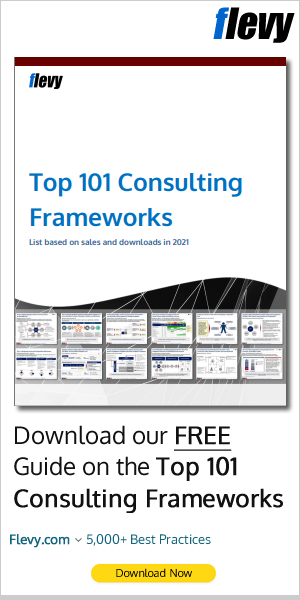